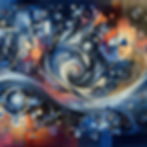
How the three-part evolutions of the web, AI, and UX herald a new technological age
We see a convergence taking place between three strands of digital technology and experience, each undergoing three phases in their evolution:
The web, moving from read -> write -> own
AI, moving from rule-based -> learning -> agentic, and
UX, moving from viewing -> interacting -> outcomes
As these strands converge, a new paradigm of technology emerges. A new paradigm, characterized by truly autonomous systems and experiences.
In this post, we explore the three-part evolution of each strand, their ultimate convergence, and the new class of products they enable.
1. Evolution of the Web: read -> write -> own
The web has gone through multiple evolutions since its inception. The early phase of the internet, now often referred to as Web 1.0, was characterized by static web pages that offered content for users to read. Information during this phase mostly flowed from content creators to users, leading to a one-way interaction whereby the user mostly consumed this static content with limited ability to interact with it. The web presented itself as an extension of traditional media like magazines, newsletters, and books. Most sites were hosted by professionals or semi-professionals. Occasionally, hobbyists might host a personal static site.
Around the mid 2000s interactive and social features started to emerge, adding the ‘write’ aspect to the web. Users were empowered to create and share their own content. The platforms that emerged included social networking, blogs, wikis, and video-sharing sites. With the democratization of content creation, an explosion of content followed, culminating today in an estimated 500 hours of video being uploaded to YouTube every minute. The way we interacted with the web became more dynamic. Sharing and collaboration became key features of the web.
With the arrival of decentralized technologies like blockchain, it became possible for users to ‘own’ their personal data, contributions, and digital assets. Through permissionless and trustless networks, users can retain increased sovereignty. Where in Web 2.0 the user is often the product of a large corporation, in Web 3.0 the user is set to regain control.
From passive consumers of content in Web 1.0, to active contributors in Web 2.0, to owners of their data and digital assets in Web 3.0, this progression signifies a move towards a more interactive, collaborative, and decentralized internet.
2. Evolution of AI: rule-based -> learning -> agentic
We argue that we can observe a similar three-part evolution in AI.
In the earliest phase of artificial intelligence (AI), roughly from the 50s to the 80s, rule-based systems gained prominence. These systems operated on a set of predefined rules and logic to make decisions. Examples include Chess computers and the first AI chatbot, Eliza. Due to the need to define rules for all possible scenarios, these systems were limited and inflexible – their creations were expensive.
A new type of AI, ‘Learning AI’ found increasing attention in the late 20th and then particularly in the early 21st century. Machine learning, and later deep learning, introduced a paradigm shift: rather than hard coding all its rules, the system could now learn information and derive its own rules from data. These systems found a wide application, from pattern recognition to predictions and speech recognition. This phase was fueled both by algorithmic advancements (backpropagation, transformers, etc.) and hardware improvements (including more computational power, specialized GPUs, and more data storage capabilities). We would argue that this phase has culminated with the arrival of large language models (LLMs).
Now, with the phenomenal rise and proliferation of LLMs, we believe the future of artificial intelligence is increasingly likely to be agentic. ‘Agentic AI’ involves AI systems that exhibit a degree of agency, autonomy, and the ability to not only make decisions but also then enact them, in complex, unpredictable environments. Agentic AI systems will often involve many AI subsystems like Natural Language Processing (NLP), computer vision, and autonomous decision making. As agentic AI systems receive new information and interact with their environment they can improve and adapt. Self-initiated actions are one of the key distinguishing features from pure AI models.
To summarize, we argue AI has evolved from rule-based systems to learning systems, and now towards agentic AI, representing increasing complexity, adaptability, and autonomy.
3. Evolution of UX: viewing -> interacting -> outcomes
Alongside the Web and AI, we argue user experience has witnessed a three-part evolution, too.
As discussed above, during the early days of the internet (known as Web 1.0) and when personal computers first became popular, most people used them mainly for looking at or reading content. Consequently, the design of websites and computer programs (user interfaces or UIs) was basic and didn't offer much room for users to interact or change things to their liking. They were mostly designed for users to read them like books or magazines. A lot of attention was given to organizing information clearly and making these websites easy to use.
As we have seen, the rise of Web 2.0 and mobile devices led to user-generated content, which led to more interaction and engagement-oriented User Experience (UX). Dynamic content, user-generated content, interactive media, and responsive UI design became the norm, all geared towards increasing user participation in an intuitive and joyful context. User journeys became a core focus of design considerations.
Now, we believe we are entering a new phase characterized by autonomous experiences. Such experiences will include products with unprecedented degrees of personalization and end-user value generation. This phase will orbit around a novel class of product that we call ‘outcomes’. Outcomes here means complex, fully-packaged value delivered to a user based on their requirements, by an agentic AI system. Real-world examples of such ‘outcomes’ have existed for decades. For example, take bespoke travel agencies: rather than writing you an itinerary, they take your requirements, select from a range of transport, accommodation, and experiences, and book and pay for those packages for you. As more and more services are digitized and made accessible, incorporating AI, it becomes possible to harness agentic AI to create software agents that deliver this kind of value across many types of industry, from finance to health and relationships. Personalization of outcomes is further increased when these products evolve to anticipate users’ desires and make them a reality before you even have to ask for them. As personalization increases, it will be more important than ever that users have ownership of the systems that create outcomes for them.
The UX evolution – from enabling viewing, to interacting, to entire outcomes – will yield increasingly sophisticated and personalized digital experiences.
The apex product
At Valory, we believe that this convergence of trends in UX, Web, and AI is not merely academic, but ushers in a new age of digital experiences: co-owned agentic AI systems that create desirable outcomes for us. Olas is already doing just that.
If you want to get a glimpse of this future, then run your co-ownable AI agent today.